The Many Facets of D&I
Advancing Technology Exposes Insurers to Bias Risk
Certain data, such as credit scores, are considered inherently biased and can have unintended consequences, according to a panel of industry leaders and analysts.
Insurers are relying on data, analytics, algorithms and digital tools to improve the industry's accessibility and efficiency. But those practices are coming under increasing scrutiny for possibly introducing unintended bias.
A panel of insurance industry experts met with AM Best TV to discuss how those exposures may be occurring. The panel included Doug Ommen, Iowa insurance commissioner and chair of the National Association of Insurance Commissioners' Big Data and Artificial Intelligence Working Group; Jake Appel, chief strategist for O'Neil Risk Consulting & Algorithmic Auditing; and Nick Frank, partner in the consulting firm of Simon-Kucher & Partners. Following is an edited transcript of the discussion.
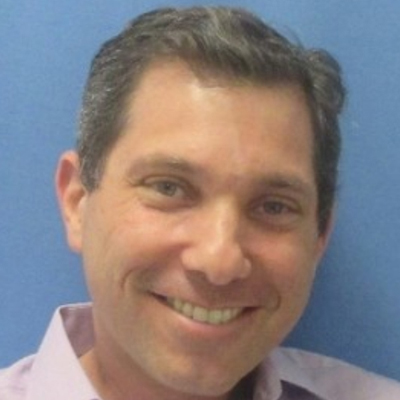
Insurance is the original data and AI business of predicting risk. That’s what AI in data analytics does: It allows you to predict things. The problem with some of the data and some of the predictions that are made is if the data is inherently biased.
Nick Frank
Simon-Kucher & Partners
Are we putting too much emphasis on data, sometimes to the detriment of the consumer?
Frank: I would say yes. I think that if you use the wrong type of data that is inherently biased because of historical reasons, that data, such as credit scores, can have unintended consequences and unintended biases especially depending on how the data is weighed.
What should we do about that?
Frank: I think the smartest thing and the thing that most companies that I work with use [is] segmentation or pricing or packaging or underwriting. They try to have data more closely [related] to the activities that they're trying to create insights [for]—for example, user-based insurance.
If you think about credit scores as an example, it was a proxy for theoretically how responsible someone is or how well they behave. Instead of using a proxy, using UBI data or user-based insurance, their braking, their driving habits, things of that nature actually have a stronger correlation to the potential risk and the potential losses for proper pricing and tiering purposes.
Do you think that we should somehow be looking at data and analytics a little bit differently?
Frank: Insurance is the original data and AI business of predicting risk. That's what AI in data analytics does: It allows you to predict things. The problem with some of the data and some of the predictions that are made is if the data is inherently biased.
For example, what happened traditionally in the U.S.'s past—related to redlining and certain properties not being able to be covered by insurance or mortgages—created depressed areas which then created a ZIP code having a worse rating for your potential insurance than another ZIP code.
We assume that data and analytics are the answer to just about everything when it comes to insurance. Perhaps, sometimes it's giving us the wrong answer. Would that be an accurate statement?
Appel: I certainly agree. Those techniques do sometimes lead us in the wrong direction. I would say it's less an issue with the data science techniques themselves and more that we sometimes ask the wrong question or aim it at the wrong thing.
An example of that from the insurance world is Optum Health Insurance, which had a program to refer certain complicated medical patients to a concierge program that would help them coordinate care. They only had a limited number of spots for that program. They used an AI predictive algorithm to figure out which of their policyholders would benefit the most from this program.
They trained that algorithm to identify the patients for whom concierge service would create the biggest cost savings. That makes sense. Thinking about developing that program and how to make it most useful from a bottom-line perspective—it's like saying, “How can we save the most dollars on medical costs?” It seems like, to a first approximation, a reasonable approach.
What the data scientists didn't recognize in doing that was that, historically, different patients with the same level of medical complexity are treated differently. In particular, African-American patients are undertreated for any given level of sickness compared to white patients.
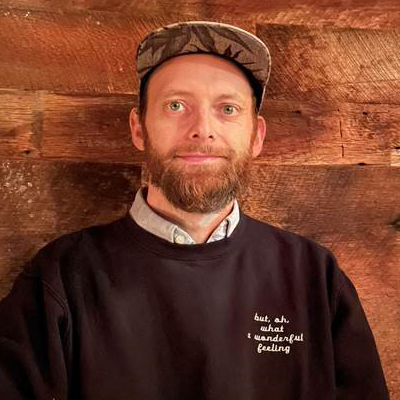
We audit algorithms particularly for issues relating to ethics, bias and discrimination. For us, an audit fundamentally starts by asking this question: For whom does this fail?
Jake Appel
O’Neil Risk Consulting & Algorithmic Auditing
Your company does something called algorithm audits. What exactly is that?
Appel: We audit algorithms particularly for issues relating to ethics, bias and discrimination. For us, an audit fundamentally starts by asking this question: For whom does this fail? By this, we mean a particular algorithm or model being deployed in a particular context. We insist on fixing a specific use case.
Once we've got that use case, we identify the stakeholders—that is, anybody who has a stake in how that algorithm performs. We interview them to hear their concerns, to elicit their concerns. Once we have a sense of their concerns, then we can create statistical tests, monitors and other ways of measuring whether those concerns are being realized in the way the algorithm's being deployed. To the extent they are being realized, we can come up with ways of mitigating those issues.
Who's getting these audits and why are they getting them?
Appel: Most of our clients are private companies in regulated industries. There are some public agencies and nonprofits. For the most part, it's private companies. Why are they doing it? Occasionally, we get a mission-driven client who simply wants to do the right thing. I wish there were more of those. More often, it's because companies are scared.
In particular, they're scared that they may be running afoul of a particular law or regulation. In that case, it's often the legal department or the compliance department that call us up. In other cases, they're simply scared of a bad headline. There are so many areas where algorithms operate but where there are not obvious compliance guidelines, laws, rules, etc.
What are you learning from these audits?
Appel: I would say we're learning three big lessons. The first lesson is that there are virtually no incentives for any individual company to choose a reasonable or high rule definition of fairness. For that reason, we feel, as a company, that it's a case for regulatory or possibly coordinated action by companies. People need to establish the guardrails.
If it's going to be anything other than the lowest common denominator, it's got to come from outside individual companies. That's the first lesson. The second lesson we've learned is that companies are really afraid of breaking particular laws and particular rules.
We do some other work aside from our audit-work assisting; for instance, attorneys general investigating suspected cases of algorithmic misbehavior, let's say. Those investigations usually boil down to this: Did the algorithm break this particular law?
What we're seeing is that the best low-hanging fruit for enforcement and real action around these issues is translating existing laws into specific rules that can be applied to algorithms. That's the second lesson.
Finally, the third lesson is, we're finding out, again and again, something that we've known from the literature for a long time, which is that it's not sufficient to worry about the data going in. We have to look at the outcomes—the decisions or scores produced by the model—to assess whether it's fair.
The NAIC recently conducted a survey as it pertains to data and analytics. Can you tell us a little bit about that survey?
Ommen: The charge or another way of saying it is the assignment that's been given to the big data and artificial intelligence working group is to gather facts that are related to the topics the panelists have been talking about, including information concerning the extent of AI system use by the insurers so that we and other NAIC workstreams can develop.
Then, we'll openly debate well-informed regulatory policy. We did meet on July 9 and developed a strategy for moving forward with our investigation. Since that time, a smaller investigative group has met a number of times as we've put together our plan.
What we decided is to issue a fairly comprehensive survey of over 180 national private-passenger auto carriers to conduct an analysis on that particular segment of the market to evaluate both the use and—as other panelists have mentioned—what I would describe as the governance associated with the use of AI in big data to take a careful look at gathering that information, as I mentioned, in order to better be able to debate the public policy aspects of the issues.
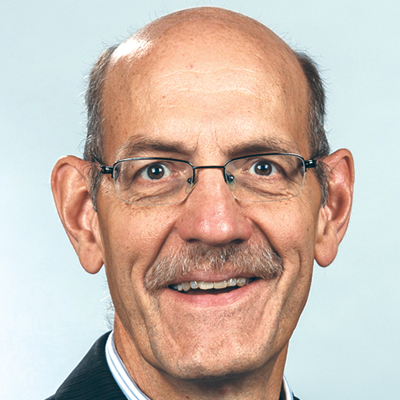
The reality is that we are a diverse country. Our educational systems have become more diverse. Are we where we want to be? No.
Doug Ommen
Iowa Insurance Division
Is there so much attention, however, given to data and analytics that we're not seeing the forest for the trees? The forest, of course, being risk-based insurance.
Ommen: Again, these are very sensitive issues. Part of our challenge, as we've moved away from much more public rating and underwriting systems, is by using data we are creating this question, these concerns. Is the data measuring race? Is it measuring economic disparities that you might be able to [address] because of historical differences that relate somehow to race?
Is it simply measuring risk disparities that can also be correlated to race? In other words, the world of 2021 is a very different world than the world when I was in high school. The reality is that we are a diverse country. Our educational systems have become more diverse. Are we where we want to be? No.
What do we do about all this data and analytics?
Ommen: I've been chairing this working group now for a number of years. We've had many public discussions. Probably the most important thing is, do not stop. We got behind. There are many who say we are still behind. I would say that the insurance business is continuing to evolve and change. Many of these technologies are incredibly valuable in order to properly price risk.
Do we have to take great care when it comes to the assignment of risk?
Appel: It made me think of the fiasco in the last few months or another tweetstorm maybe around Lemonade insurance and personalized assessment of risk that used some components of facial analysis. I'm not an expert on this exact tweetstorm.
Suffice to say, Lemonade was using some way of assessing risk that maybe had some validity to it but which, on its face, was hard for someone to understand why, I don't know, the way my face appeared in the particular little video snippet that I submitted or something might have anything to do with my risk level.
There's been such a push to rely on data and analytics in the insurance industry. What should the industry be doing so as not to marginalize further some of its most vulnerable consumers?
Frank: The thing we all do as professionals in insurance with datasets, and AI, and analytics, is we want to know what data doesn't just correlate but is causal to a particular risk or outcome—making sure that your data-like number of citations or previous accidents is strongly correlated to the risks that we underwrite. As long as people are focused on trying to remove biases, that's an important thing.
Where do we go from here?
Frank: It's through the project teams who are building the algorithms like my teams, being very focused on having open dialogues about whether the data that they're using or the insights that come out of that data are going to negatively impact one group or the other.
Appel: The goal of algorithmic auditing as we're doing it now is to bring to the forefront those ethical dilemmas and questions. Is it OK to be charging a female doctor slightly more or less likely to underwrite her because she represents a larger risk?
Ommen: By and large, many Americans are very willing to accept the risk associated with being classified as male or classified as female. You can look at the life insurance market as an example. My wife and I may be the same age by date of birth but because of our gender, we're not the same age based on mortality.
What we have to do is take great care as we look at those things that, as described earlier, are morally acceptable or socially acceptable and compare that to what is economically acceptable for a 63-year-old male like myself looking to buy insurance.
AM Best TV
Go to www.bestreview.com/d-i to watch the full lineup of programs in The Many Facets of D&I.